Birds have evolved to fly like the wind and adapt to any adversity in the air. For example, they can sense sudden changes in their surroundings, including the onset of sudden turbulence. They can quickly adjust to stay safe. Aircraft engineers want to give their vehicles the same sense of skills to adjust on the fly.
AI-Trained Aerial Vehicles
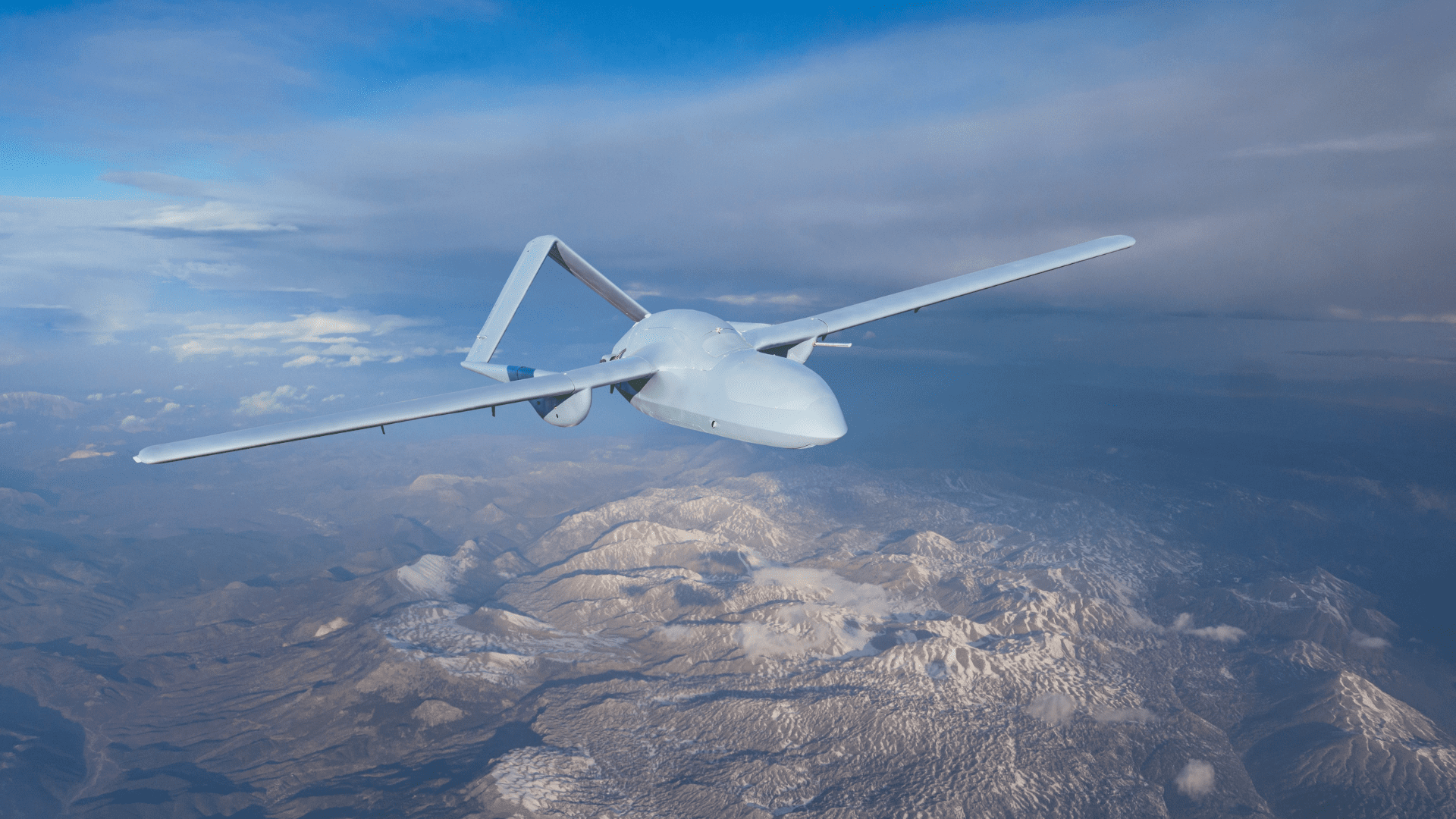
A team of researchers from Caltech’s Center for Autonomous Systems and Technologies (CAST) and Nvidia is one step closer to that capability. In a recent study, the researchers described a control strategy they developed for unmanned aerial vehicles (UAVs) called FALCON (Fourier Adaptive Learning and CONtrol).
The strategy uses a form of artificial intelligence called reinforcement learning. Using reinforced learning, the UAV adaptively learns how turbulent wind can change over time. Then, it uses that knowledge to control the UAV based on what it is experiencing in real time.
Mory Gharib is the Hans W. Liepmann Professor of Aeronautics and Medical Engineering, the Booth-Kresa Leadership Chair of CAST, and the author of the new paper. “Spontaneous turbulence has major consequences for everything from civilian flights to drones,” said Gharib. He explains that climate change and weather events that cause this type of turbulence are rising.
“Extreme turbulence also arises at the interface between two different shear flows—for example, when high-speed winds meet stagnation around a tall building,” he said. “Therefore, UAVs in urban settings need to be able to compensate for such sudden changes.” According to the researchers, FALCON gives the vehicles notice when the turbulence is coming so they can make necessary adjustments.
Put to the Test
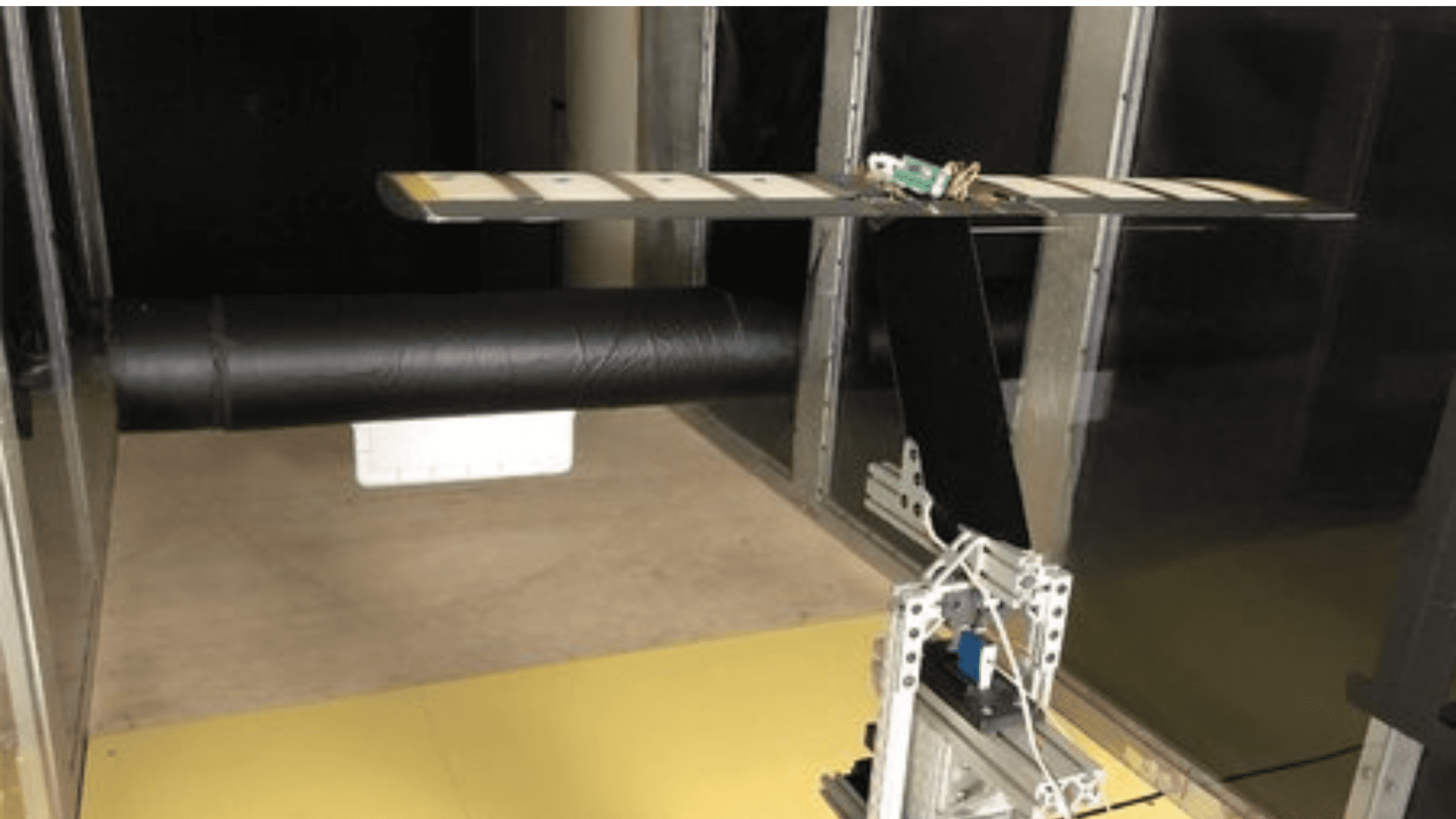
To test the effectiveness of the FALCON strategy, the researchers created an extremely challenging test setup in the John W. Lucas Wind Tunnel at Caltech. During the study, a fully equipped airfoil wing system represented a UAV. They outfitted it with pressure sensors and control surfaces that could adjust things like the system’s altitude and yaw. Then, they positioned a large cylinder with a moveable attachment in the wind tunnel. When wind flowed over the cylinder, it replicated turbulence as it reached the airfoil.
“Training a reinforcement learning algorithm in a physical turbulent environment presents all kinds of unique challenges,” said Peter I. Renn, a co-lead author of the paper who is now a quantitative strategist at Virtu Financial. “We couldn’t rely on perfectly clean signals or simplified flow simulations, and everything had to be done in real-time.”
According to the findings, the FALCON-assisted system stabilized itself in this extreme environment after about nine minutes.
“With each new observation, the program gets better because it has more information,” said one of the study’s authors, Anima Anandkumar.
“The future really depends on how powerful the software gets in terms of needing less and less training,” Gharib added. “Quick adaptation is going to be the challenge, and we are going to push, push, push.”
In the future, the researchers envision UAVs and passenger planes having the ability to share sensed and learned information about conditions with each other. Having the capability of sharing information about hazardous conditions enhances the safety of aerial travel.
“I believe that’s going to happen,” said Gharib. “Otherwise, things will get pretty dangerous as extreme weather events increase in frequency.”